Introduction
The advent of quantum computing marks a revolutionary shift in the field of technology, and its impact on artificial intelligence (AI) applications is poised to be profound. This article delves into the intriguing dynamics of how quantum computing will affect AI applications, exploring the symbiotic relationship between these two groundbreaking technologies.
Quantum Computing: A Brief Overview
Quantum computing stands at the forefront of technological innovation, poised to revolutionize computation as we know it. This brief overview delves into the core concepts of quantum computing, exploring qubits, quantum gates, quantum algorithms, and the notion of quantum supremacy.
Quantum Bits (Qubits)
The qubit is the basic component of a quantum computer and it is a quantum analogue to the bit. However unlike classic bits which can only exist in the states of 0 or 1, qubits are in superposition of both states due to the principles of quantum mechanics. It holds this characteristic that multiplies the computational capability by means of the parallel application of large volumes of data. In the table below, classical bits are compared to a qubit.
Property | Classical Bits | Qubits |
---|---|---|
State | 0 or 1 | Superposition |
Information Unit | Binary | Quantum |
Processing Power | Limited | Exponential |
Quantum Gates
Quantum gates serve as the basic units of the quantum circuits, the same way a classical logic gate forms the circuit of classical computing. They maneuver in the quantum states with a help of qubits so they can do computation operations. There are arrays of quantum gates, depending on the function. The table below overviews the main quantum gates, showing their different functions.
Quantum Gate | Operation |
---|---|
Hadamard | Creates superposition |
Pauli (X, Y, Z) | Rotations around the X, Y, and Z axes |
CNOT | Entangles two qubits, conditional NOT |
Quantum Algorithms
Quantum algorithms use quantum physics to enhance the processing power in finding solutions of problems which are at a rate of exponential speedup from that of classical algorithms. Shor’s algorithm and Grover’s algorithm are the significant cases of these computation procedures. These algorithms apply quantum parallelism and interference that lead to abilities of the quantum computers that are otherwise impractical to classical computers. The table below looks at the diversities between classical and quantum algorithms.
Algorithm | Classical | Quantum |
---|---|---|
Shor’s Algorithm | Exponential time | Polynomial time |
Grover’s Algorithm | Quadratic time | Square root time |
Quantum Supremacy
Quantum supremacy symbolizes an extraordinary achievement of a quantum computer performing some tasks incomparable to the performance of classical supercomputers. In the year 2019, Google’s Sycamore processor demonstrated quantum supremacy by performing a task in less than 3 minutes compared to the approximately 10,000 years that the world’s fastest classical computer would require. This development marks the advent of a brand new era in terms of computational power and most likely it helps to solve unforeseeable complex problems.
Amplifying AI with Quantum Computing
Quantum computing is set to enhance AI applications in several key ways:
Data Processing and Analysis
The fact that a quantum computer is capable of computing with large datasets with a new level of efficiency and speed is provided. The traditional computers become overwhelmed by the complexity of large-scale data analysis through calculating power bottlenecks. Nevertheless, quantum computers are distinct in terms of their ability to process more than one data at a time using qubits or quantum bits. Developing AI technologies based on a quantum computing approach therefore allows them to promptly process in-depth data sets, draw strategic information, and adapt accordingly in real time. Here are the main advantages:
- Speed: Quantum computers are capable of computing data exponentially more than classical computers can which leads to quicker decision-making in machine learning;
- Scalability: Quantum algorithms scale proportionally to the dataset size, allowing AI systems to tackle immense data sets that are otherwise problematic for traditional AI systems;
- Complexity: Quantum computing, which gives the capacity to solve sophisticated big data analysis problems exceeding classical computing abilities, makes possible additional AI functions, such as natural language processing, image recognition, and predictive analytics.
Optimization Problems
Artificial intelligence programs relieve optimization issues such as route planning in logistics or resource allocation in supply chain management. Quantum annealing and variational algorithms being the cornerstone of quantum enjoying a quantum leap in tackling complex optimization problems. The quantum annealers, such as the ones from the D-Wave Systems, are specially tailored to solving the combinatorial optimization tasks and are acclaimed for their big advances from the classical optimization methods. Here are the main advantages:
- Efficiency: Certain quantum optimization algorithms such as annealing procedure become better in solving large-scale optimization problems than classical techniques and consequently computing consumes less time and resources;
- Accuracy: Quantum computing also reduces the probability of getting caught in the local optima which means that the AI applications would be near-perfect in solving computationally extensive optimization problems for highly complicated tasks;
- Versatility: With quantum optimization, the specific applications for AI can be designed to address diverse industries’ needs in resource allocation, scheduling, and network routing allowing companies to achieve a higher performance level overall and potentially outperform their competitors.
Machine Learning Enhancements
Quantum computing leverages up the machine learning effectiveness by speeding up training information, raising models’ accuracy, and developing quantum inspired algorithms. Quantum-proliferated machine learning algorithms lend front seat to foundational quantum physics principles such as superposition and entanglement to enhance feature extraction, pattern recognition and predictive modeling. Here are the main advantages:
- Speedup: With quantum machine learning algorithms, training and inference operations run faster, and models are able to learn from information through AI models more and more quickly;
- Generalization: Quantum-inspired algorithms enable AI models to develop a generalization capability which in turn allows them to perform far better on unseen data and prevents them from overfitting;
- Complexity Handling: Quantum computing is significantly superior in the evaluation of high-dimensional input spaces and thus can encompass more complex patterns compared to classical logic.
Security
In contrast, quantum computing introduces new means for higher security guarantees for cryptography and encryption. The lattice-based key distribution and quantum key distribution (QKD) protocols which are resistant to quantum attacks are quantum-proof mechanisms. These protocols guarantee the protection of high-level data in AI applications. Key advantages include:
- Post-Quantum Security: Quantum-resistant encryption algorithms defend AI systems to quantum computers’ future potential threat security, that is why data confidentiality and integrity long term are ensured;
- Unforgeability: Quantum key distribution protocols allow for secure communication channels between AI modules by keeping completely private data during its transfer;
- Resilience: Quantum-resistant security solutions harden AI stacks against new malevolent factors using shields which in turn safeguards the vigor and the integrity of AI systems across different industries.
Real-World Examples and Data
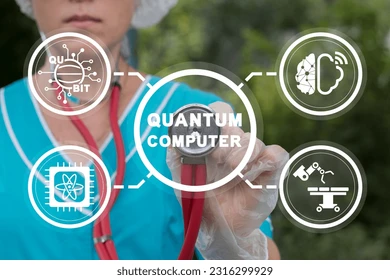
Quantum computing has emerged as a groundbreaking technology with immense potential to revolutionize various industries. When coupled with artificial intelligence (AI), quantum computing unlocks new possibilities for solving complex problems with unprecedented speed and accuracy. Let’s explore real-world examples where the synergy between quantum computing and AI is making significant strides:
Healthcare
Quantum computing has ushered in a new era of innovation in healthcare by significantly enhancing AI capabilities in various domains.
- Diagnostic Tools: Quantum computing enables the development of faster and more accurate diagnostic tools by harnessing the principles of quantum mechanics. These tools empower AI algorithms to analyze vast amounts of medical data swiftly and accurately, leading to early detection of diseases and improved patient outcomes;
- Medical Imaging: Quantum-enhanced AI algorithms can process medical imaging data more efficiently, leading to higher resolution images and better detection of anomalies. This advancement is crucial in improving diagnostic accuracy and enabling personalized treatment plans;
- Drug Discovery: Quantum computing accelerates the drug discovery process by simulating molecular interactions with unprecedented accuracy. AI algorithms leverage these simulations to identify potential drug candidates more efficiently, leading to the development of novel therapies for various diseases.
Quantum computing combined with AI has enabled researchers to analyze genomic data more comprehensively, leading to the discovery of personalized treatments for cancer patients.
Finance
In the financial sector, the integration of quantum computing and AI is driving significant advancements in risk assessment and fraud detection.
- Risk Assessment: Quantum-enhanced AI models can analyze vast datasets and complex risk factors with unparalleled speed and precision. This capability enables financial institutions to assess risks more accurately, leading to better-informed decision-making and optimized investment strategies;
- Fraud Detection: Quantum computing enhances the effectiveness of AI algorithms in detecting fraudulent activities by analyzing patterns and anomalies in financial transactions in real-time. This proactive approach helps mitigate financial losses and strengthens cybersecurity measures.
Leading financial institutions are leveraging quantum computing-powered AI systems to detect fraudulent transactions with greater accuracy, thereby safeguarding against financial crimes.
Environmental Modeling
Quantum computing plays a pivotal role in advancing AI applications for environmental modeling, particularly in climate science.
- Climate Modeling: Quantum computing enables the simulation of complex environmental systems with unprecedented accuracy and speed. AI algorithms leverage these simulations to analyze climate data, predict environmental changes, and assess the impact of human activities on the ecosystem;
- Natural Disaster Prediction: Quantum-enhanced AI models can process vast amounts of data from various sources, including satellite imagery and sensor networks, to predict natural disasters such as hurricanes, earthquakes, and wildfires more accurately. This early warning system helps communities prepare and mitigate the impact of these catastrophic events.
Scientists are using quantum computing-powered AI models to simulate climate scenarios and evaluate the effectiveness of mitigation strategies for addressing climate change.
Quantum Computing’s Impact on Specific AI Domains
Quantum computing represents a paradigm shift in the field of artificial intelligence (AI), unlocking new capabilities and enhancing existing applications across various domains. Let’s delve into how quantum computing is revolutionizing specific AI domains:
Enhanced Natural Language Processing (NLP)
Quantum computing plays a pivotal role in advancing natural language processing (NLP) capabilities, enabling AI systems to understand and process human language more naturally and efficiently.
- Chatbots and Virtual Assistants: Quantum-enhanced AI algorithms can analyze linguistic patterns and context more effectively, leading to the development of chatbots and virtual assistants that engage in more meaningful and human-like interactions. These systems can comprehend nuances in language, understand user intent, and generate responses with greater accuracy;
- Efficient Language Understanding: Quantum computing enables AI models to process vast amounts of textual data in parallel, leading to faster language understanding and sentiment analysis. This enhanced efficiency is particularly valuable in applications such as customer service, where quick and accurate responses are essential for user satisfaction.
Breakthroughs in Autonomous Vehicles
Quantum computing is driving breakthroughs in AI algorithms used in autonomous vehicles, enhancing their decision-making capabilities and safety features.
- Faster Decision-Making: Quantum-enhanced AI algorithms can process sensor data from autonomous vehicles more efficiently, enabling faster decision-making in dynamic environments. This capability is crucial for ensuring the safety of passengers and pedestrians by allowing vehicles to react swiftly to changing road conditions and traffic patterns;
- Enhanced Safety Features: Quantum computing enables AI systems to perform more sophisticated simulations and predictive modeling, leading to the development of advanced safety features in autonomous vehicles. These features include collision avoidance systems, adaptive cruise control, and predictive maintenance algorithms, which collectively enhance the overall safety and reliability of autonomous transportation systems.
Revolutionizing AI in Gaming
In the gaming industry, quantum computing is revolutionizing AI capabilities, enabling the creation of more immersive and responsive gaming environments.
- Realistic Simulation: Quantum-enhanced AI algorithms can simulate complex game environments with unprecedented accuracy, leading to more realistic gameplay experiences. These simulations can model intricate physics interactions, dynamic environments, and sophisticated non-player character behaviors, enhancing the overall immersion and realism of gaming worlds;
- Responsive Gameplay: Quantum computing enables AI systems to process player inputs and adapt game mechanics in real-time, leading to more responsive and personalized gameplay experiences. This capability allows games to dynamically adjust difficulty levels, tailor content to individual player preferences, and provide engaging and challenging experiences for players of all skill levels.
The Future Landscape
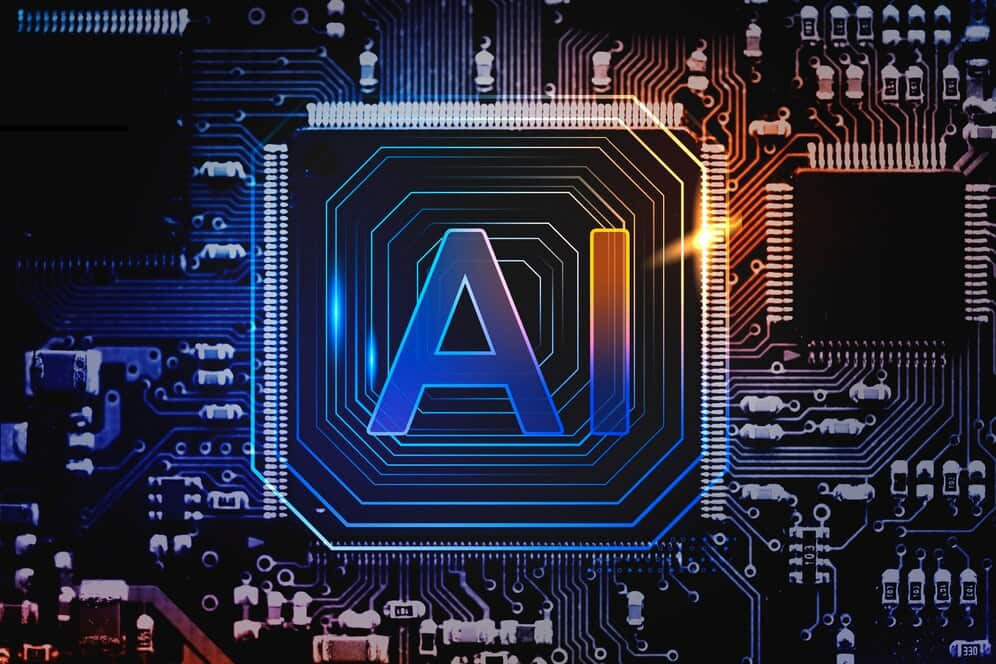
As we look ahead, the convergence of quantum computing and artificial intelligence (AI) holds immense promise for shaping the future landscape of technology. However, despite the remarkable benefits, there are also potential limitations and challenges that must be addressed to realize the full potential of this synergy.
The Potential Limitations
While the synergy between quantum computing and AI presents unprecedented opportunities, several challenges hinder the widespread adoption of this transformative technology:
- Quantum Hardware: The development of robust quantum hardware remains a significant hurdle. Quantum computers require precise control of quantum bits (qubits) and low error rates to perform complex computations reliably. Current quantum hardware is susceptible to noise and decoherence, limiting the scalability and practicality of quantum computing for large-scale AI applications;
- Specialized Quantum Algorithms: Traditional AI algorithms must be adapted and optimized for quantum computing architectures. Developing specialized quantum algorithms capable of exploiting the unique properties of quantum mechanics is essential for realizing the full potential of quantum-AI systems. However, designing and implementing these algorithms pose technical challenges and require interdisciplinary expertise in quantum physics and computer science.
The Road Ahead
Despite the challenges, the integration of quantum computing and AI is still in its nascent stages, and the road ahead is filled with opportunities for transformative innovation:
- Technology Advancements: Continued advancements in quantum hardware and software are expected to overcome existing limitations and unlock new capabilities for quantum-AI systems. Research efforts are focused on improving qubit coherence times, reducing error rates, and scaling up the number of qubits to build more powerful quantum computers suitable for AI applications;
- Interdisciplinary Collaboration: Collaboration between researchers, scientists, and industry stakeholders across multiple disciplines is crucial for driving innovation in quantum computing and AI. Interdisciplinary research initiatives aim to bridge the gap between quantum physics, computer science, and AI, fostering the development of novel algorithms, tools, and methodologies for quantum-AI integration;
- Sector-wide Impact: As technology continues to advance, the potential for quantum-AI-driven innovations extends across various sectors, including healthcare, finance, cybersecurity, and environmental science. Quantum-AI systems have the potential to revolutionize drug discovery, optimize financial portfolios, strengthen cybersecurity defenses, and model complex environmental systems with unprecedented accuracy.
Conclusion
The question of how quantum computing will affect AI applications opens the door to a future brimming with possibilities. This fusion is set to revolutionize how we approach problems, offering solutions with speed and efficiency previously unimaginable. As we step into this new era, the synergy between quantum computing and AI promises to transform the technological landscape, redefining what is possible.
FAQ
Quantum computing uses qubits that can exist in multiple states simultaneously, unlike classical bits that are either 0 or 1.
Quantum computing enhances AI’s data processing capabilities, optimizes complex problem-solving, and accelerates machine learning processes.
Healthcare, finance, environmental science, autonomous vehicles, and gaming are among the industries that stand to gain significantly.
The main challenges include the need for more advanced quantum hardware and the development of quantum algorithms suited for AI applications.
While some applications are already in use, the broader impact will become more evident as quantum computing technology matures over the next decade.